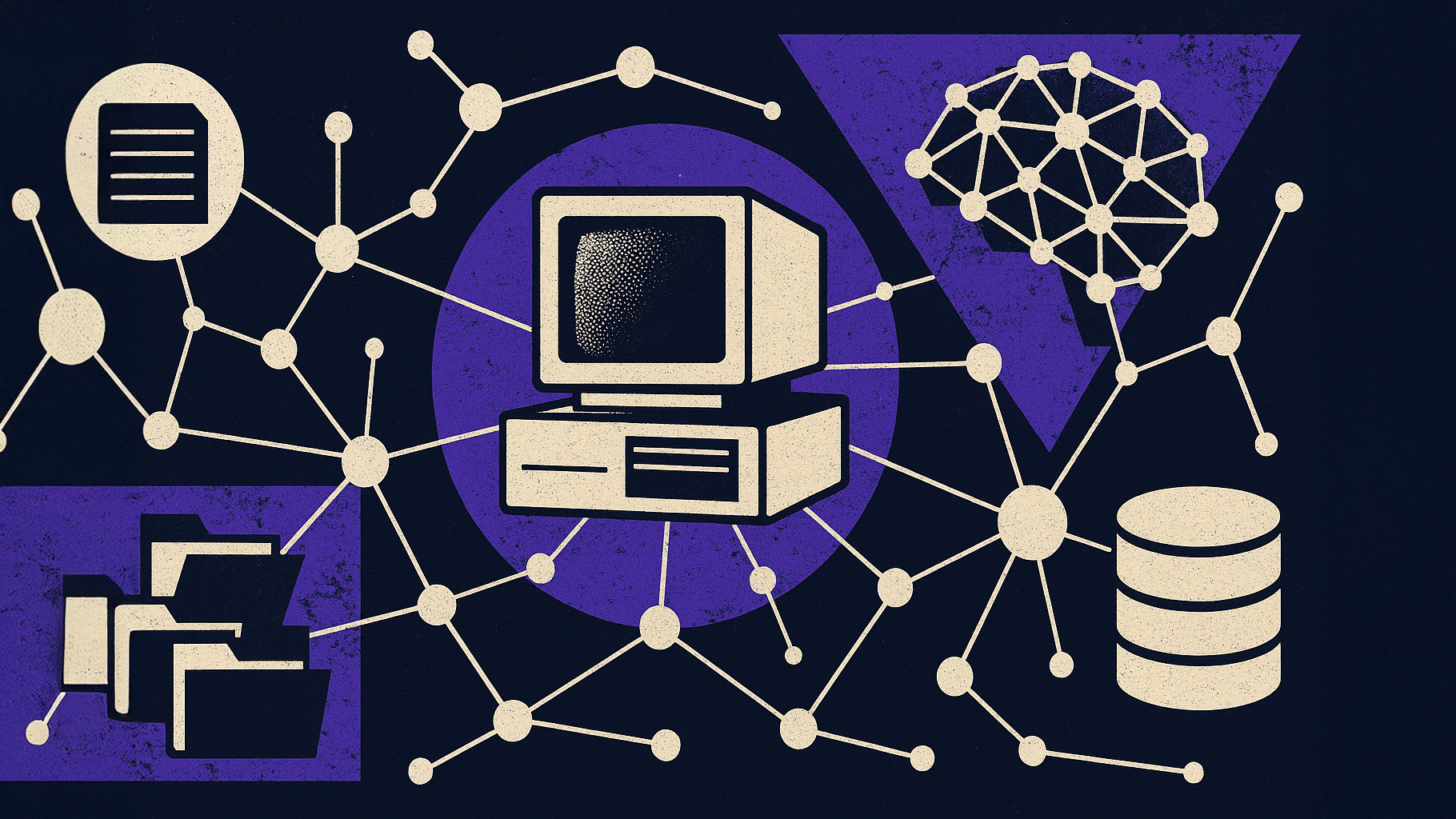
Unifying Enterprise Data for Effective Generative AI
Explore the transformative power of unifying enterprise data to enhance generative AI capabilities across cloud platforms.
The Need for Data Unification in Modern Enterprises
In today's data-driven world, enterprises generate and collect vast amounts of data from various sources. This data can be structured, semi-structured, or unstructured, and it often resides in disparate systems across the organization. The need to unify this data is more critical than ever, particularly as companies look to leverage generative AI to gain actionable insights and maintain a competitive edge.
Unifying enterprise data allows organizations to break down silos, ensuring that data is accessible and usable across different departments and applications. This unified approach not only improves data quality and consistency but also enhances the ability to perform complex analytics and machine learning tasks. By combining data from various sources, enterprises can create a comprehensive view of their operations, leading to better decision-making and more effective AI models.
Challenges of Fragmented Data Silos
One of the most significant challenges enterprises face is the fragmentation of data into silos. These silos often result from legacy systems, different data management practices, and the rapid adoption of new technologies. Fragmented data silos can hinder the ability to gain a holistic view of the organization, leading to inefficiencies and missed opportunities.
Data silos can also pose significant obstacles to the implementation of generative AI. AI models require vast amounts of high-quality data to be effective. When data is fragmented, it is challenging to ensure that AI models have access to the comprehensive and accurate data they need. This fragmentation can lead to biased or incomplete models, reducing the overall effectiveness of AI initiatives.
Integrating Structured, Semi-Structured, and Unstructured Data
To build a robust data foundation for generative AI, enterprises must integrate structured, semi-structured, and unstructured data. Structured data, such as databases and spreadsheets, is highly organized and easily searchable. Semi-structured data, like JSON and XML files, has some organizational properties but lacks a fixed schema. Unstructured data, such as text, images, and videos, does not have a predefined format and is often the most challenging to manage.
Integrating these different types of data requires a flexible and scalable data architecture. Enterprises should adopt a data lakehouse model, which combines the best features of data lakes and data warehouses. This model allows organizations to store, process, and analyze all types of data in a unified environment. By leveraging modern data integration tools and platforms, enterprises can ensure that their data is accessible and usable for generative AI applications.
Best Practices for Metadata Management
Effective metadata management is crucial for unifying enterprise data and ensuring its usability. Metadata provides context and information about the data, making it easier to search, discover, and understand. Proper metadata management practices help maintain data quality, improve data governance, and enhance the overall usability of data.
Enterprises should establish a centralized metadata repository that captures and stores metadata from all data sources. This repository should include information about data lineage, quality, and usage. Additionally, organizations should implement automated metadata management tools that can continuously update and maintain metadata as data changes. These tools can help ensure that metadata remains accurate and up-to-date, supporting the effective use of data for generative AI.
Leveraging Open Data Formats for Seamless Integration
Using open data formats is essential for seamless data integration across different systems and platforms. Open formats, such as CSV, JSON, and Apache Parquet, are widely supported and can be easily read and processed by various tools and applications. By adopting open data formats, enterprises can ensure that their data is accessible and interoperable, reducing the complexity of data integration.
Open data formats also facilitate data sharing and collaboration within and outside the organization. For example, JSON is a lightweight and flexible format that is ideal for exchanging data between systems and applications. Apache Parquet, on the other hand, is optimized for analytical workloads and can efficiently store large volumes of data. By leveraging these open formats, enterprises can create a more flexible and scalable data architecture that supports generative AI initiatives.
Building a Scalable Multimodal Data Lakehouse
A scalable multimodal data lakehouse is the foundation for effective generative AI. This architecture combines the storage capabilities of data lakes with the performance and management features of data warehouses. It allows organizations to store and process structured, semi-structured, and unstructured data in a unified environment, enabling comprehensive analytics and AI applications.
To build a scalable multimodal data lakehouse, enterprises should focus on the following key components:
- Unified Storage Layer: Implement a storage layer that can handle various data types and formats, ensuring that all data is stored in a centralized repository.
- Data Processing Engine: Use a powerful data processing engine that can efficiently process large volumes of data and support different types of workloads, such as batch processing and real-time analytics.
- Metadata Management: Establish a robust metadata management system that captures and maintains metadata for all data sources, ensuring data quality and governance.
- Open Data Formats: Adopt open data formats to facilitate data integration and interoperability across different systems and platforms.
- Security and Compliance: Implement strong security measures and compliance policies to protect sensitive data and ensure regulatory compliance.
By focusing on these components, enterprises can build a scalable multimodal data lakehouse that supports generative AI and drives business value.
Ensuring Data Security and Compliance
As enterprises unify their data, ensuring data security and compliance becomes increasingly important. With the growing volume of data and the complexity of data architectures, organizations must implement robust security measures to protect sensitive information and comply with regulatory requirements.
Key practices for ensuring data security and compliance include:
- Access Controls: Implement fine-grained access controls to restrict access to sensitive data and ensure that only authorized users can access specific data sets.
- Data Encryption: Use encryption to protect data at rest and in transit, ensuring that sensitive information remains secure.
- Data Masking: Apply data masking techniques to obfuscate sensitive data, reducing the risk of unauthorized access and data breaches.
- Regulatory Compliance: Implement policies and procedures to ensure compliance with relevant regulations, such as GDPR, CCPA, and HIPAA. Regularly audit data practices to identify and address compliance gaps.
- Monitoring and Auditing: Continuously monitor data access and usage, and conduct regular audits to detect and respond to security incidents and ensure compliance with policies.
By implementing these practices, enterprises can protect their data and ensure compliance with regulatory requirements, supporting the safe and effective use of generative AI.
Real-World Benefits of Unified Data for Generative AI
Unifying enterprise data provides numerous benefits for generative AI applications. By breaking down data silos and integrating structured, semi-structured, and unstructured data, organizations can create a comprehensive view of their operations and unlock new insights.
Some of the key benefits of unified data for generative AI include:
- Improved Model Accuracy: Access to comprehensive and high-quality data improves the accuracy and effectiveness of AI models, leading to better predictions and insights.
- Enhanced Decision-Making: Unified data provides a holistic view of the organization, enabling more informed and data-driven decision-making.
- Increased Efficiency: Streamlined data integration and processing reduce the time and effort required to manage and analyze data, leading to increased operational efficiency.
- Innovation and Competitive Advantage: Unified data enables organizations to develop innovative AI applications and maintain a competitive edge in the market.
- Better Customer Experiences: Generative AI powered by unified data can provide personalized and contextually relevant experiences for customers, enhancing customer satisfaction and loyalty.
By unifying their data, enterprises can fully leverage the power of generative AI to drive business value and achieve their strategic goals.