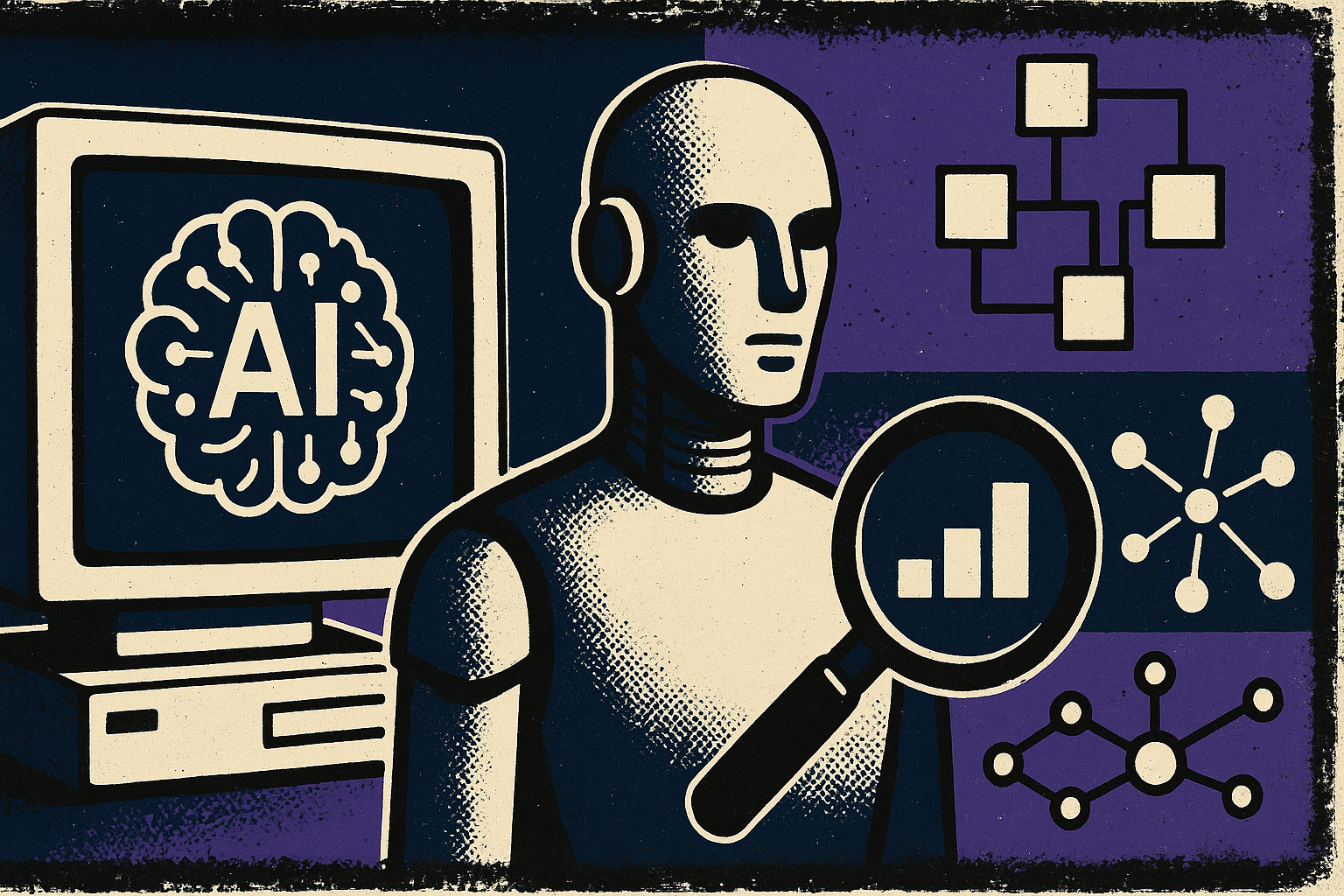
Boosting Productivity with Generative AI Agents in Data Management
The Role of Generative AI Agents in Modern Data Management
In today's rapidly evolving digital landscape, data-driven organizations are constantly seeking innovative ways to enhance productivity and streamline their operations. One of the most promising advancements in this realm is the integration of generative AI agents. These intelligent systems are designed to simplify complex tasks, automate routine processes, and provide deeper insights into data, making them indispensable tools for modern data management.
Generative AI agents leverage advanced machine learning algorithms to perform a variety of functions, from generating natural language responses to complex data queries, to visualizing data in more intuitive ways. By automating these tasks, AI agents free up valuable time for data scientists, analysts, and other stakeholders, allowing them to focus on higher-level strategic activities.
Simplifying Natural Language Data Queries with AI
One of the most significant ways generative AI agents can boost productivity is by simplifying natural language data queries. Traditionally, extracting meaningful insights from large datasets required proficiency in complex query languages like SQL. This often posed a barrier for non-technical users who needed to access and analyze data.
Generative AI agents overcome this challenge by enabling users to interact with data using natural language. Users can pose questions in plain English, and the AI agent translates these queries into the appropriate data retrieval commands. This not only democratizes data access but also speeds up the process of obtaining insights, as users no longer need to rely on specialized knowledge or wait for assistance from technical teams.
Enhancing Data Visualization for Better Insights
Data visualization is a critical component of data analysis, as it transforms raw data into comprehensible visual formats that highlight trends, patterns, and anomalies. Generative AI agents enhance this process by automatically generating sophisticated visualizations based on user queries and the underlying data.
For instance, an AI agent can automatically create charts, graphs, and dashboards that are tailored to the specific needs of the user. These visualizations can be dynamically updated as new data is ingested, ensuring that stakeholders always have access to the most current information. Additionally, AI-driven visualizations can incorporate advanced features like predictive analytics, which provide foresight into future trends based on historical data.
Conversational Analytics: A New Approach to Data Interaction
Conversational analytics represents a paradigm shift in how users interact with data. By combining natural language processing (NLP) with generative AI, conversational analytics allows users to engage in a dialogue with their data, making the interaction more intuitive and accessible.
Generative AI agents facilitate this by interpreting user queries, providing contextually relevant responses, and even suggesting follow-up questions to delve deeper into the data. This conversational approach not only enhances user engagement but also leads to more thorough and meaningful analyses, as users can iteratively explore data without the need for extensive training or technical expertise.
Streamlining Model Deployment for Non-Technical Users
Deploying machine learning models has traditionally been a complex and resource-intensive process, often requiring specialized skills and significant time investments. Generative AI agents simplify this process by automating many of the steps involved in model deployment, making it accessible even to non-technical users.
AI agents can guide users through the entire model deployment lifecycle, from selecting the appropriate data and features to training and validating the model. They can also handle the technical aspects of deploying the model into a production environment, ensuring that it integrates seamlessly with existing systems and workflows. This streamlining of the deployment process enables organizations to quickly operationalize their AI initiatives and realize value from their data.
Real-World Use Cases of Generative AI Agents
The potential of generative AI agents is best illustrated through real-world use cases across various industries:
-
Retail: Retailers can use AI agents to analyze customer behavior, optimize inventory levels, and personalize marketing campaigns. For example, The Home Depot leverages AI to improve product search efficiency and enhance customer experience.
-
Healthcare: In the healthcare sector, AI agents assist in analyzing patient data, predicting disease outbreaks, and personalizing treatment plans. These applications not only improve patient outcomes but also increase operational efficiency.
-
Finance: Financial institutions use generative AI agents to detect fraudulent activities, assess credit risks, and provide personalized financial advice to clients. These applications help mitigate risks and enhance customer satisfaction.
-
Agriculture: Companies like Bayer Crop Science utilize AI agents to analyze environmental data, optimize crop yields, and streamline research and development processes. This leads to more sustainable and efficient agricultural practices.
Integrating AI Agents Across the Data Lifecycle
To fully realize the benefits of generative AI agents, organizations need to integrate them across the entire data lifecycle. This involves creating a cohesive data strategy that encompasses data collection, storage, processing, analysis, and visualization.
-
Data Collection: AI agents can automate the collection of data from various sources, ensuring that it is accurate, complete, and up-to-date.
-
Data Storage: Utilizing scalable and flexible data storage solutions, such as cloud-based data lakes, allows organizations to manage large volumes of data efficiently.
-
Data Processing: AI agents can preprocess data, handle data integration tasks, and ensure that the data is clean and ready for analysis.
-
Data Analysis: By leveraging AI-driven analytics platforms, organizations can perform complex analyses and generate actionable insights.
-
Data Visualization: Integrating AI agents with visualization tools enables the creation of dynamic and interactive dashboards that provide real-time insights.
-
Model Deployment: AI agents streamline the deployment of machine learning models, allowing organizations to operationalize their AI initiatives quickly.
Future Trends and Opportunities in AI-Driven Data Management
The future of AI-driven data management is poised for exciting developments. As AI technology continues to advance, we can expect to see even more sophisticated and capable AI agents that can handle increasingly complex tasks.
-
AI-Driven Decision Making: AI agents will play a more prominent role in decision-making processes, providing real-time recommendations and insights that drive business strategies.
-
Advanced Predictive Analytics: The integration of AI with predictive analytics will enable organizations to anticipate future trends and make proactive decisions.
-
Enhanced Data Security: AI agents will help organizations enhance data security by identifying vulnerabilities, monitoring for suspicious activities, and ensuring compliance with regulations.
-
Personalized User Experiences: AI-driven data management will enable the creation of highly personalized user experiences, tailored to the specific needs and preferences of individual users.
In conclusion, generative AI agents have the potential to revolutionize data management by simplifying complex tasks, enhancing productivity, and providing deeper insights into data. By integrating AI agents across the data lifecycle, organizations can unlock new opportunities for innovation and growth. As we look to the future, the continued advancements in AI technology promise to further transform the way we manage and utilize data.